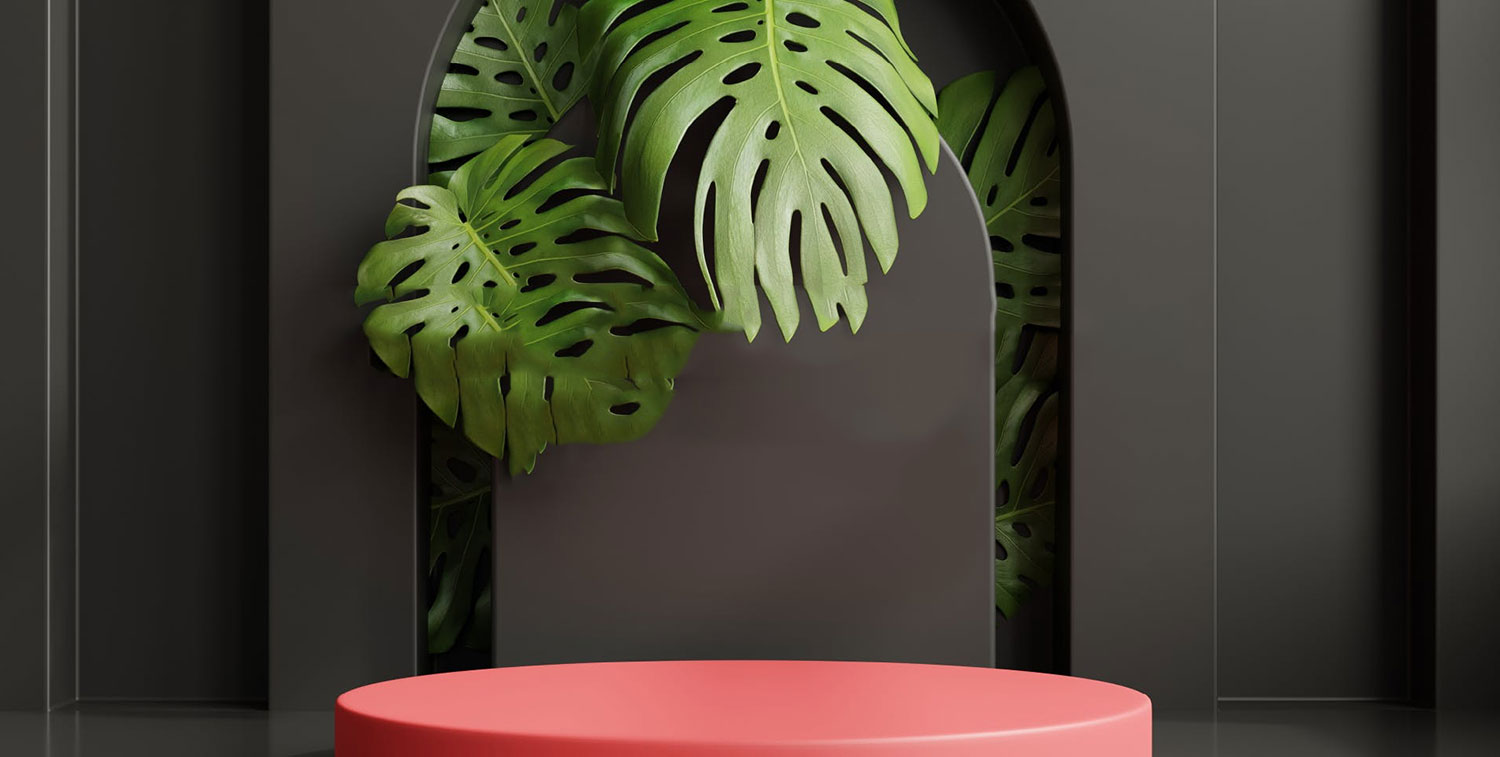
In recent times, there appears to be increasing interest in the use of generative A.I. in user experience research and design (UXD/R). Many use cases have been proposed by influential UXers. But one potential use case that is often neglected is detecting UXR bias. User experience research can be plagued by hidden biases, impacting results, and ultimately leading to products that exclude certain user groups. But what if generative AI could help?
Generative AI and Large Language Models (LLM) offer exciting possibilities, for instance in Detecting bias. Generative A.I can analyze research instruments to identify and flag potential leading questions & cultural nuances. As an Example, assuming you want to know if a given user interview question is subject to bias. You could use any existing G A.I tool or for fun build your own GPT—Research Bias GPT, better if you train your own LLM. In the example below, I used an existing G A.I. Pay attention to the prompt.
Prompt: Analyze the following survey question for potentially leading language and its impact on participants’ responses: “Would you agree that the new mobile app is much easier to use than its competitors?”
ChatGPT: The survey question “Would you agree that the new mobile app is much easier to use than its competitors?” contains leading language that can significantly influence the participants’ responses. Here’s an analysis of its components and the potential impact:
Leading Language
- Assumption of Agreement:The phrase “Would you agree that” presupposes there is a consensus or a correct view that the new mobile app is easier to use. This can pressure respondents to conform to this implied agreement, skewing the responses towards the affirmative.
- Comparative Superiority:By stating “much easier to use than its competitors,” the question assumes that the app is not only easier to use but significantly so. This introduces a bias by suggesting that the app is superior before the respondent has provided their opinion.
- Absence of Neutral Comparison:The question does not allow for a neutral or comparative evaluation of the app against its competitors without the presumption of its superiority. It doesn’t give respondents a fair chance to consider their actual experiences or preferences impartially.
Impact on Participants Responses
- Response Bias:The leading nature of the question can introduce response bias, where participants may lean towards agreeing with the statement, even if their actual opinion is different. This can result in artificially high satisfaction ratings or skewed feedback that doesn’t accurately reflect users’ experiences.
- Social Desirability Bias:Participants might answer in a way they believe is expected or desirable to the surveyor. Knowing the question suggests the app is superior, they might endorse this view to align with perceived expectations, rather than providing an honest assessment.
- Limited Insight into User Experience:The question’s framing restricts the depth of insight that can be gained about the app’s usability. It misses the opportunity to explore specific areas where the app performs well or falls short compared to competitors, leading to a less nuanced understanding of its user experience.
Recommendations for Improvement
To mitigate these issues and gather more objective and valuable feedback, the question could be rephrased to remove leading language and assumptions. For example:
- “How would you compare the ease of use of the new mobile app with its competitors?”
- “Please rate the ease of use of our mobile app compared to its competitors.”
These alternatives allow respondents to express their views without being influenced by the question’s wording, providing more reliable and actionable insights into the app’s user experience and competitive standing.